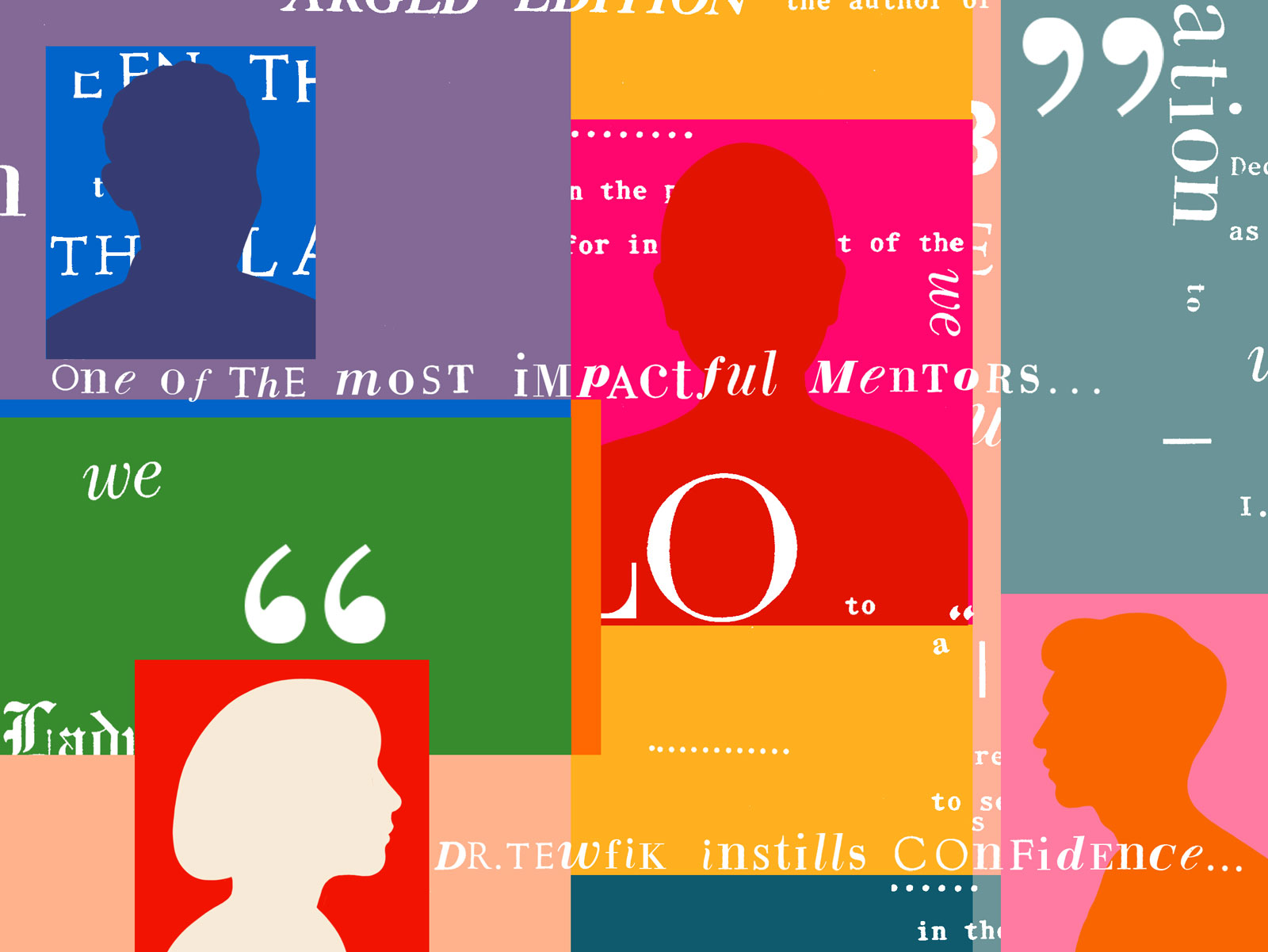
MIT Sloan Professor Relishes Role as a UROP Mentor
Basima Tewfik, who studies impostor syndrome, honored by students for her guidance and commitment to undergraduate research
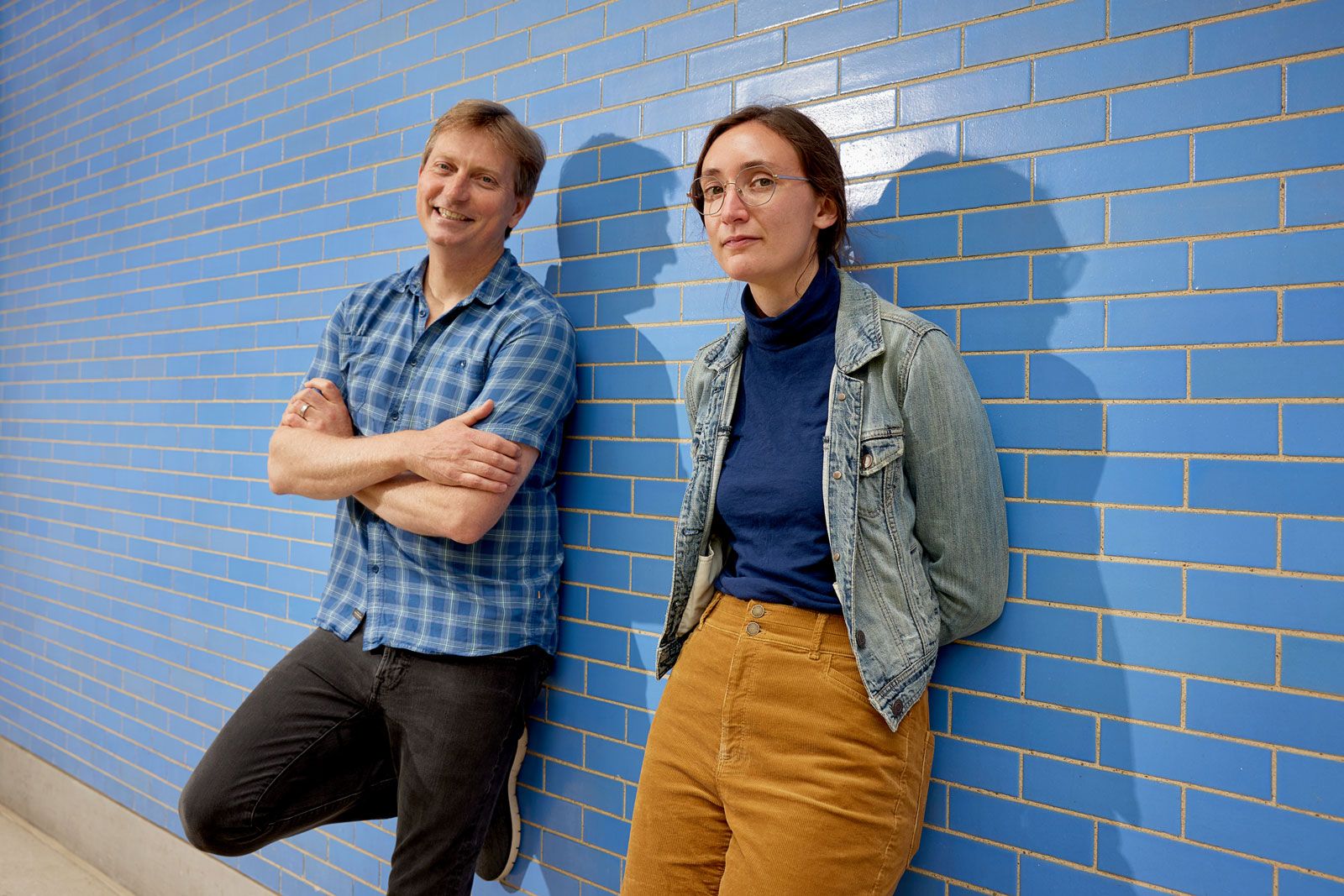
Joey Davis and Laurel Kinman: A Multidimensional Collaboration
Biology professor's support inspires PhD student and protein enthusiast to prioritize mentorship in her career
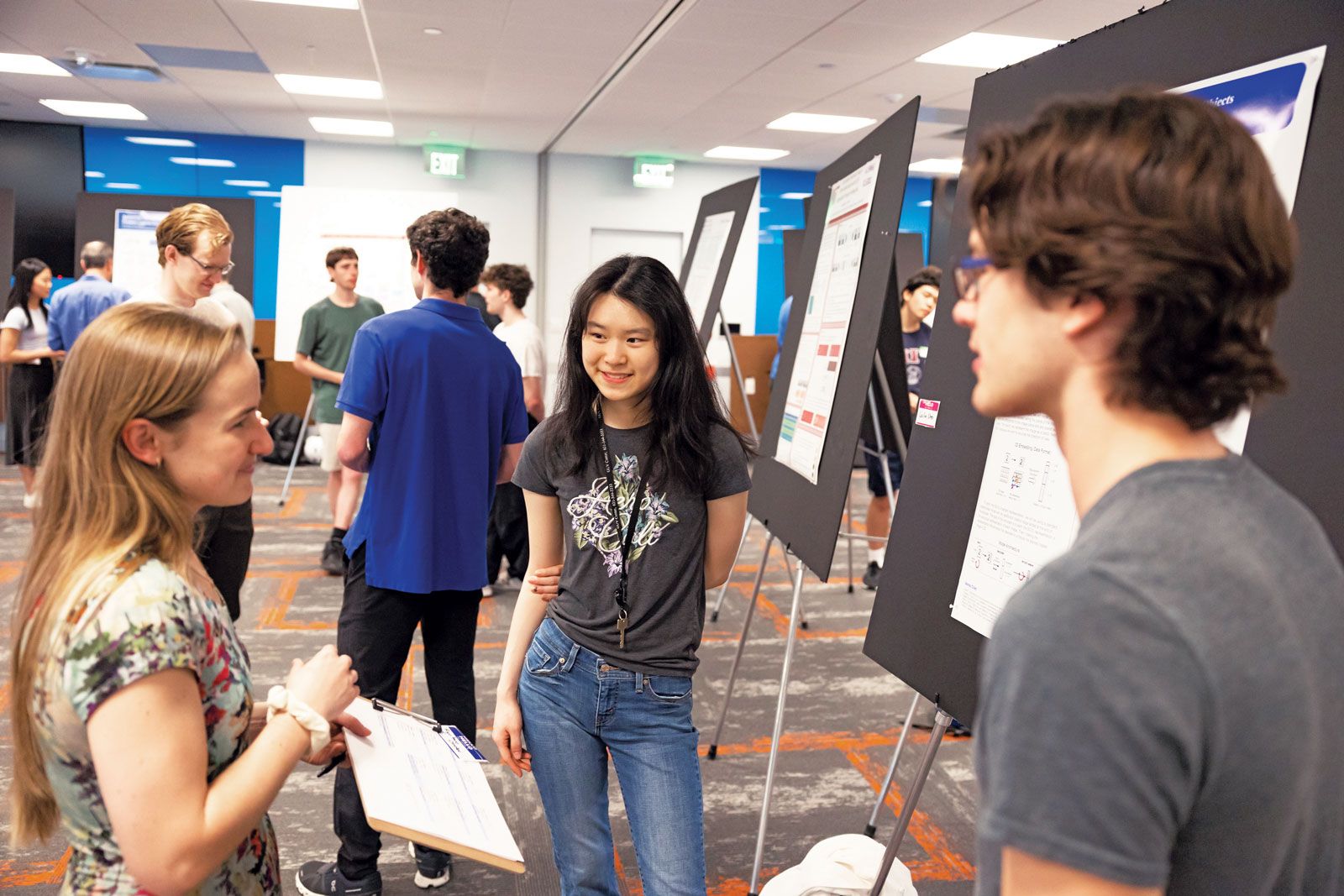
Engineers, Express Yourselves
The MIT School of Engineering Communication Lab, which turned 10 in 2023, helps students translate their work for broader impact
Mentorship in Action Across Campus
MIT's vice president for Resource Development talks about MIT’s robust mentoring landscape and how alumni and friends can play a part
Passing the Baton of Science
Hologic-funded Jay A. Stein Professorship of Biology celebrates MIT innovation across generations
A Trusted Guide Can Make All the Difference
MIT President Sally Kornbluth on mentorship
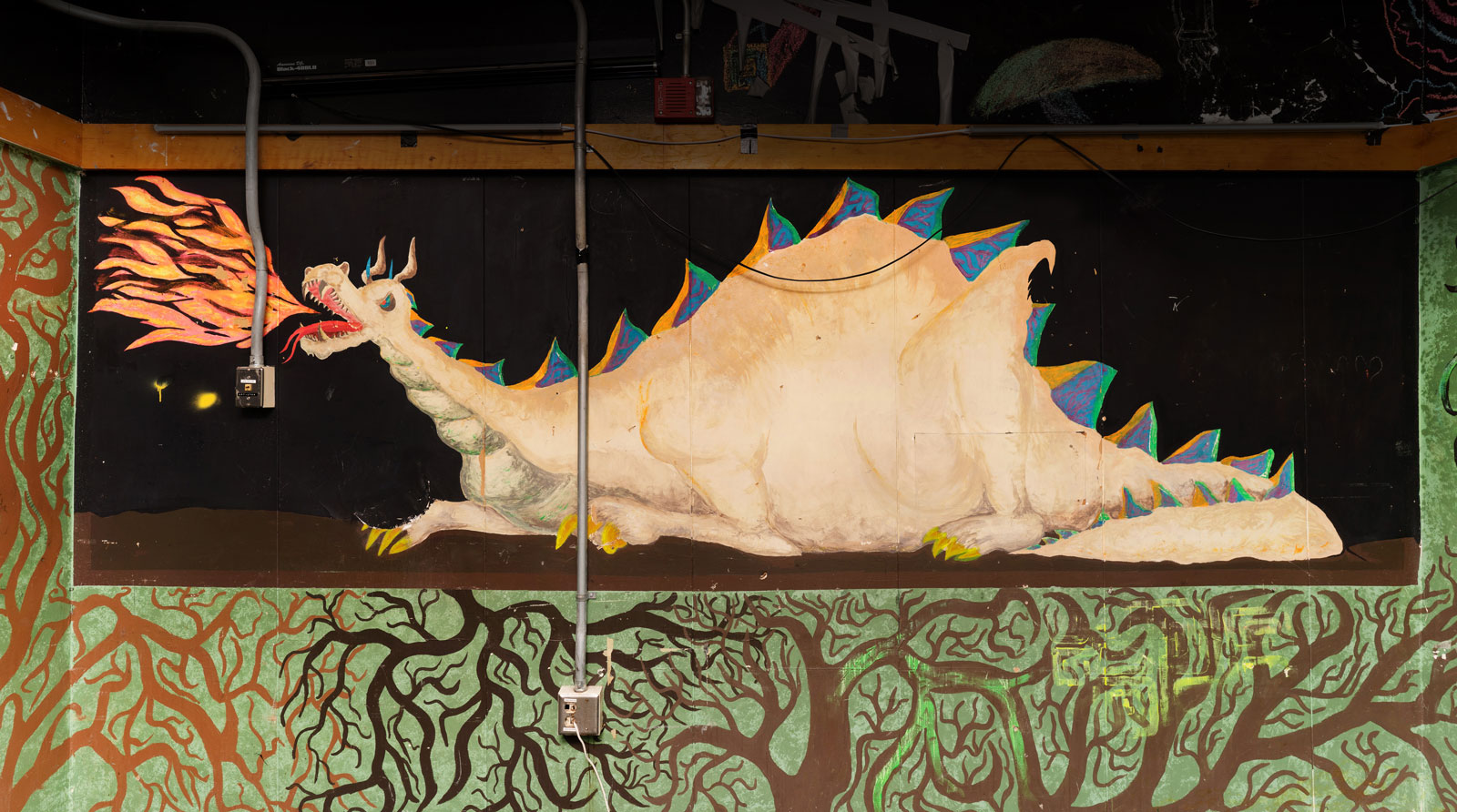
Legacy of Student Art in East Campus
Before East Campus closed in summer 2023 for renovations, a project to digitally preserve some of the most beloved student art got underway
Spotlight On: Mentorship
Your Friendly Neighborhood Mentors at MIT
Resident Peer Mentor program formalizes the peer support that has always been a hallmark of residential culture at the Institute
Designing Effective Applications
MIT graduate architecture students offer guidance to potential applicants from underrepresented groups
Yang Shao-Horn and Betar Gallant Have Positive Energy
Collaboration with Professor Shao-Horn, who works to uncover materials for next-generation batteries, cultivated Associate Professor Gallant’s intellectual potential
MIT programs provide a mentorship-paved pathway to doctoral degrees—and a potential launch pad to academia
Popular
Alumni Say Whiteman Fellowship Gave Them the Freedom to Explore
Fund supporting aspiring astrophysicists and Earth, atmospheric, and planetary scientists marks a milestone
In the Pioneering MIT Department of Economics, Researchers Turn a Scientific Lens to Policy
Economists offer insights and interventions for health care, education, and work
MIT donors contribute to creation of new Welcome Center and Earth and Environment Building plus renovation of beloved Kresge Auditorium